Supply Chain Analytics Benefits
With today’s distributed global supply chains and the continual disruptions, all stakeholders need to be tightly coordinated to ensure flexibility, resiliency and rapid product or solution time-to-market. Modern supply chain data analytics brings you end-to-end visibility into every step of your logistics network and even supports real-time demand and supply shaping. Here are the specific benefits for your organization:
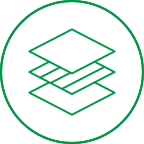
Ensure the availability of raw materials, components and/or products to increase order fulfillment and revenue
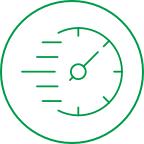
Anticipate and immediately respond to market trends and shifts within the supplier base to manage risk and proactively plan for disruption
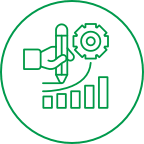
Reshape operations and embed efficiencies through the chain to lower cost of goods sold (COGS) and achieve high gross margins
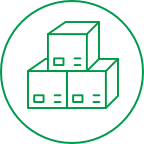
Reduce order fulfillment issues to enhance customer satisfaction and drive brand loyalty
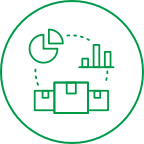
Gain a complete view of the business, uncovering accurate and actionable insights in real -time planning, sourcing, logistics and warehousing and aftermarket
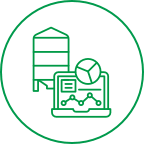
Enhance Environmental, Social & Governance (ESG) by unifying data across enterprise silos to make smarter and more transparent supplier decisions