I received a lot of questions after posting my data pipelines article about the mechanics of who creates and maintains them – more specifically, whether it’s the responsibility of the folks in data engineering or should it be left to the data scientists. The confusion is understandable when you examine job descriptions, because it’s common to see the phrase “must be able to build data pipelines.” Therefore, this post describes how I define the difference.
Which Department and What Are Their Objectives
Let’s first examine the role of the data engineer and where they work. The job title has gradually emerged in recent years and has generally replaced the older titles of “integration engineer” or “ETL developer.” Consequently, it’s a job that reports into central IT and focuses on developing the architecture, infrastructure and processes for designing, developing and maintaining enterprise-wide data pipelines.
The data scientist, on the other hand, is someone who most likely reports into a business unit and is responsible for implementing pipeline procedures to clean, wrangle, model and analyze data. Consequently, although both roles are responsible for acquiring data in a usable format, the ultimate goals are considerably different.

Data Engineering Responsibilities
Data engineers deal with raw data from applications, machines and systems. The data is typically structured or semi-structured, might not be validated and could contain anomalies, such as missing records or system-specific field values. Consequently, data engineers need to recommend and implement ways to improve data reliability, deliverability efficiency and quality, so that data sets are ready for data science consumption. Data engineers also create pipelines to reliably deliver data for other use cases, such as data migration, data warehouse ingestion and application integration.
Data Scientists' Responsibilities
Data scientists will usually get data that has passed the “first round” of cleaning and manipulation from data engineering, which they then use to feed their analytics applications, machine learning projects and statistical predictive models. However, data scientists also use their pipelines to augment that data with industry research, demographic information and behavioral data to answer pressing business questions.

Although there is some overlap in skillsets, the two roles are distinct. The data engineer has skills best suited for working with database systems, data APIs, ETL/ELT solutions, and will be involved in data modeling and maintaining data warehouses, whereas the data scientist has experience with statistics, math and machine learning for predictive models.
Languages, Software, Skills and Tools
Given we mentioned skill overlap, let’s now examine the differences in skillsets, languages, tools and software that both roles use. The languages, software, tooling and infrastructure used by data engineers runs the gamut of Enterprise IT. As we mentioned earlier, that’s the traditional trove of data tools like SQL and ELT. Increasingly, knowledge of public cloud infrastructure solutions from Amazon, Google and Microsoft is now considered mandatory for the modern data engineer. Suffice it to say, many a data engineer uses Qlik Data Integration as a core component to architect their data pipelines.
Data scientists will make use of languages such as R, Python, Julia and Scala to build models. The most popular tools, however, are Python and R. When you’re working with Python and R for data science, the languages will most often resort to opensource libraries, such as Pandas and NumPy.
Finally, we can’t leave this data science skills discussion without covering data visualization and storytelling, Although the data scientist role might focus on using Jupyter Notebooks with Python’s matplotlib, many turn to Qlik Data Analytics for enterprise-scale business intelligence and analytics visualizations, too.
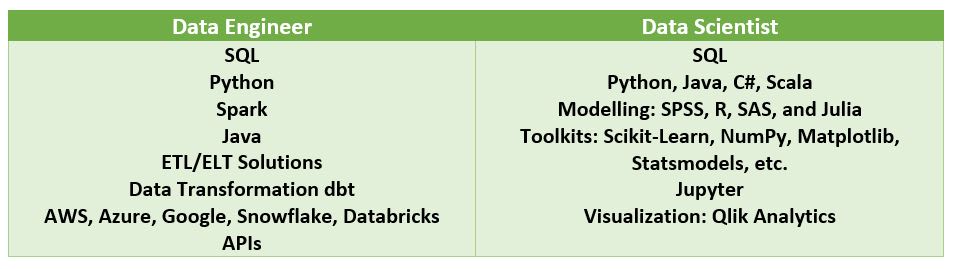
Salaries and Outlook
Now, this is the section you’re all waiting for. How do salaries compare? It’s true that the data scientist role has been in massive demand for a few years, but recently the temperature seems to have cooled a little. U.S. News & World Report’s 2021 job survey still lists Data Scientist as the eighth best job in the United States. And Glassdoor lists the median salary as approximately $114,00. Not at all shabby!
Not to be outdone, data engineering is in strong demand, too. A quick search of LinkedIn highlights over 200,000 available jobs worldwide. Again, if we check Glassdoor, the average data engineer’s salary in the United States is about $110,000. That’s only slightly lower than that of one the top 10 most desirable jobs!

Conclusion
We could argue that the “data scientist bubble” is about to burst, but there’s no denying that the demand for data expertise is strong, with a positive outlook for the immediate future. However, one thing is certain. Your prospects look good whether you choose data engineering or data science.
Confused over differences between #dataengineers & #datascientists? @Qlik's @cbearman gives you all the answers - and also compares #salaries.
In this article:
Data Integration